Generative AI (GenAI) is extremely promising, but achieving tangible results is more complex than the hype suggests. Keir Regan-Alexander, architect and founder of creative consultancy, Arka Works, highlights the challenges of implementing GenAI and offers practical strategies for professional practice
Have you noticed how AI is often written about in two dramatically different ways? a) it’s a silver bullet or b) it’s a scam. – On the one hand, it’s presented as a Frankensteinian invention that is changing the employment landscape for many knowledge workers.
On the other, people are becoming exasperated by hyped-up claims and over-promises being pushed by “corporate grifters”.
But what if neither extreme is wholly true and GenAI proves to be … c) worth the effort and much liked by the employees who use it in the real-world?
This technology is beguiling because it presents itself as friendly and simple. Ask a question and, like popping corn, it comes noisily to life. This sweet, surface level experience is why there is such a widespread conceit on LinkedIn that using Generative AI to solve all your business problems is easy.
Here’s the formula:
You post an eye-catching image and add the hook “I just solved [insert challenging task] in 5 minutes. RIP [insert profession] 🚀”
People see these posts and they conclude that a whole host of other ideas must therefore also be possible. This is the peak of inflated expectations.
They try to emulate the idea at work, only to quickly discover that the claim was only “sort of” true. When real-world constraints and quality standards are applied to the recipe, the method falls short in some critical way.
- Cue feeling disheartened.
- Cue labelling Generative AI as all hype and writing it off for anything useful.
- Cue pulling up the drawbridge of curiosity and deciding we don’t need to be laser-focused on AI after all.
The trough of disillusionment is reached, and a microcosmic version of the hype cycle is complete.
As we approach two years since GPT-3 went mainstream, we remain at the beginning of the very first innings for GenAI and it’s not productive to try and rush to definitive conclusions about what it all means just yet.
It’s also not productive to spread claims that AI is easy to do well; it’s not.
Getting high-quality repeatable results across your org using AI is hard.
Adding yet more software processes to your stack is hard.
Remembering you can do something differently when you’ve been doing it the same way for years, is hard.
The human in the loop
The prospect of a mature AI adoption landscape across industry wide settings – where complete workflows are delivered end-to-end appears at present to be a long way off. Not least because very few existing organisations have their project and operational data structured and prepared for such change.
But while regular businesses are not really ready, this is what large AI companies are planning for:
“OpenAI recently unveiled a five-level classification system to track progress toward artificial general intelligence (AGI) and have suggested that their next-generation models (GPT-5 and beyond) will be Level 2, which they call “Reasoners.” This level represents AI systems capable of problem-solving tasks on par with a human of doctorate-level education. The subsequent levels include “Agents” (Level 3), which can perform multi-day tasks on behalf of users, “Innovators” (Level 4) that can generate new innovations, and finally “Organisations” (Level 5), i.e. AI systems capable of performing the work of entire businesses. As we progress through these levels, the potential applications and impacts of AI will expand dramatically.” Stephen Hunter, Buckminster AI.
The “Level 4 Innovators” moment appears to be the point at which things will start to feel very different and this projection suggests we are 4-5 major development cycles from it.
While I believe such end-to-end workflows are more probable than not in the coming years, I’m doubtful that widespread automated workflows and decision-making without human oversight at critical steps would be a desirable outcome for anyone, even if it were possible. Dramatic shifts in technology over very short time periods can be ruthlessly inhumane when driven by purely utilitarian priorities – just look back at the Industrial Revolution and what became of the Luddite movement for reference.
Indeed, the “human in the loop” has been essential to every successful implementation of GenAI that I’ve seen in professional practice. Any high value professional task cannot happen without sound judgement, discretion and (seldom mentioned) good taste. People also take responsibility for outcomes – as Ted Chiang points out, the human creative mind expresses “creative intention” at every moment.
Until we see evidence that traditional “Knowledge Work” businesses can thrive and compete without this essential ingredient, then GenAI’s job will be to provide scaffolding that helps to prop up what we already do, rather than re-build it entirely.
One process at a time
In the last two years, the hype around AI has risen to unreasonable levels and every software product has been slathered in AI that no one asked for and that rarely works as desired.
But rather than trying to reinvent the wheel with wholesale departmental and budget changes caused by implementing a million new AI apps or hiring a team of software developers, my recent work with professional practice suggests that you’ll likely find it more impactful to refine just one humble spoke of the wheel at a time and to build momentum with incremental but lasting adaptations that support what you already do well.
When you focus on smaller, yet pivotal seeds of contribution from AI and put it in the many hands of your team, rather than mandating prescribed use from “on high”, you can strike a powerful balance: boosting your existing way of working while maintaining individual control, sound judgement and freedom over each productive step. Pretty soon, you will see new and varied species of work evolving across the office.
These adjustment to ‘chunks’ of work need to be done with vocal and transparent engagement about cultural alignment with this new technology.
The formation of an ethical framework for responsible use within the business -that sets guardrails for adoption – is also needed alongside new quality measures to keep standards from slipping, which is always a risk when you make something faster and easier.
You also need a programme for new skills training so that you can equip your team with the resources to go forth and explore for themselves. If Knowledge Work is going to change in the coming years, providing new skills and tools as broadly as you can seems the fair thing to do.
Find this article plus many more in the Sept / Oct 2024 Edition of AEC Magazine
👉 Subscribe FREE here 👈
Proving product-market-fit
If you’re doing it right, the productive benefits of AI will be felt by the business but largely flow via the employees who directly use it – employees may find they achieve more in the same time, to a higher level of quality and even with a greater sense of enjoyment in their work.
This is how I find it and I know many more who increasingly feel the same. Anecdotally, I know many employees now hold personal licences to various consumer-facing GenAI tools that they choose to pay for out of their own pocket each month because they bring such high levels of utility and improve their working lives directly.
That’s one of the purest examples of “product-market fit” that you will find, and it wouldn’t be happening unless people had worked out how to really drive value from these new techniques. Imagine paying for software you use for work out of your own pocket, just to improve your working life.
In these cases, mostly they don’t tell their employers – and I know this because people discuss it openly.
It’s possible that many leaders are simply turning a blind eye because it works for them to. Or this is an outcome caused by a kneejerk AI policy that was written over a year ago calling for the total prohibition of AI on company equipment. In these cases, policies are commonly being ignored and this is the worse outcome of all because then you have uncontrolled use, no privacy controls, likely data leakages of GDPR, NDA and commercially sensitive information out of the business.
Where to focus
Many hundreds of new software products have been marketed to professionals in the last couple of years – lists upon lists of “must have” new names and logos.
I have tested many and I like a number of them. But for every new and well-built tool there are many more in the ‘vapourware’ category that fail to effectively solve a real problem for practices or that duplicate something another tool has already done better.
Moreover, the sheer scale of new gadgets and techniques can cause paralysis in businesses who get caught in perpetual “test-mode” and become unable to take any meaningful action at all. The ever growing size of these lists doesn’t help either – it’s a never-ending task.
To keep things simple and to focus on what is really important there are in my mind two foundational technologies that require our special and ongoing attention with each new release of development.
- Image models (Diffusion models like SDXL & Flux), these can also now process video.
- Text models (Large Language Models like GPT-4o & Claude 3.5), these can also now process numerical data.
These two areas alone are enormously deep and novel fields of learning. I recommend getting familiar with how to access and make use of these tools as directly as you can in their “raw” form, i.e. don’t become too reliant on easy-to-use wrappers that do many things for you but ultimately reduce your control – you will find that many of the apps’ features you’ve seen are possible by focusing on the raw ingredients and with only a couple of low cost subscriptions needed.
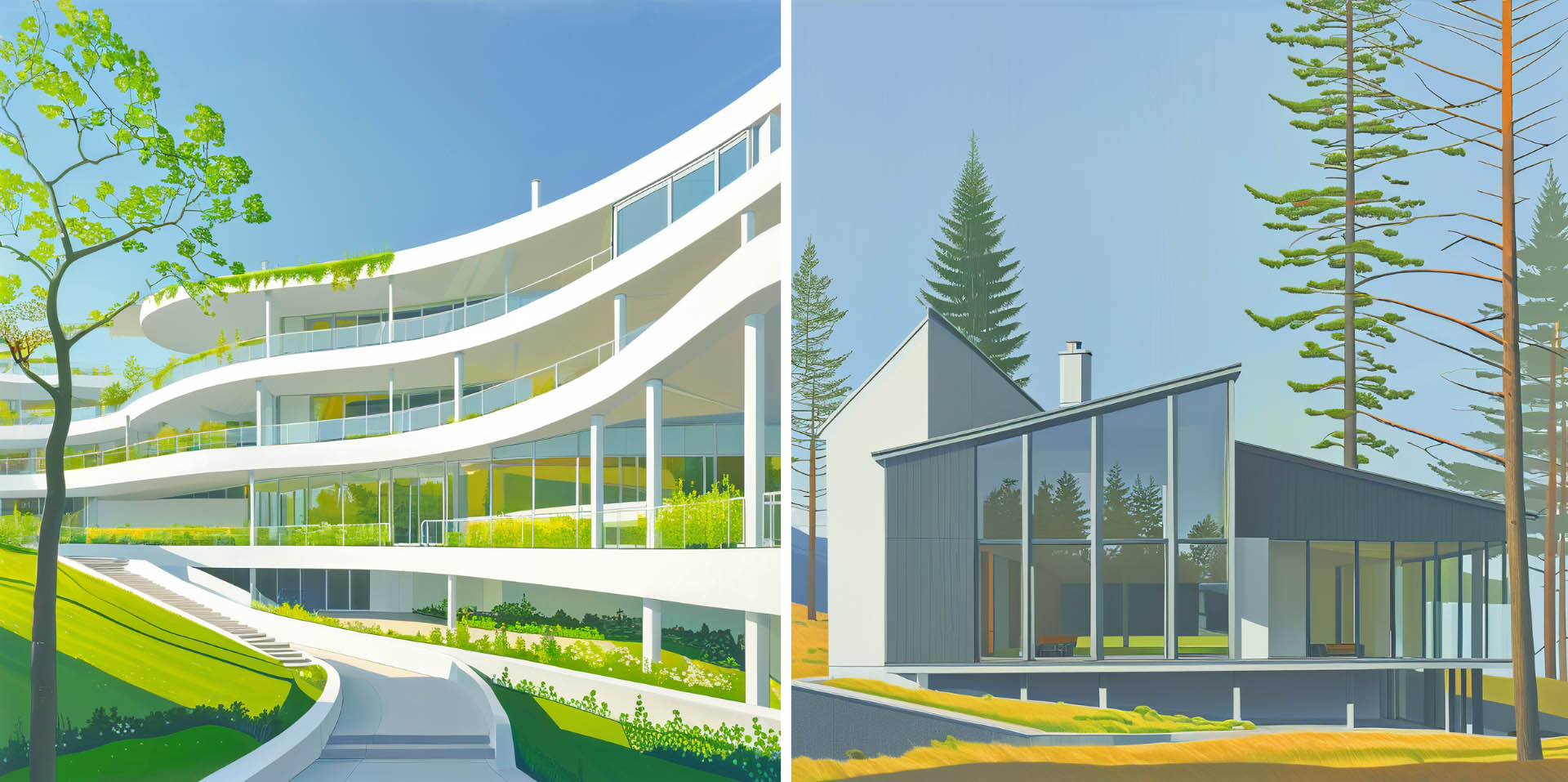
Image work in practice
My background is in design, and I love to use image models for visual concepts.
I don’t find these tools can solve multistep problems in one shot – instead, I curate their use over smaller discrete chunks of controlled taskwork and weave the whole thing together using a number of well-known tools that I would already be using in traditional practice.
To give you a better sense of what I mean, in the table below you can see some practical examples from the last month at Arka Works.
While doing these chunks of work with teams, we’re exercising our own professional and creative judgments at each step.
What you’ll notice when looking at these approaches is that they are quite complex procedures requiring a high degree of control and aesthetic judgement. Also, that the whole process is being carefully curated by the designer, which is why some people prove to be particularly gifted at working with diffusion models by following their intuition and others are less so – if the results were just the case of clicking buttons in the right order, this wouldn’t be true.
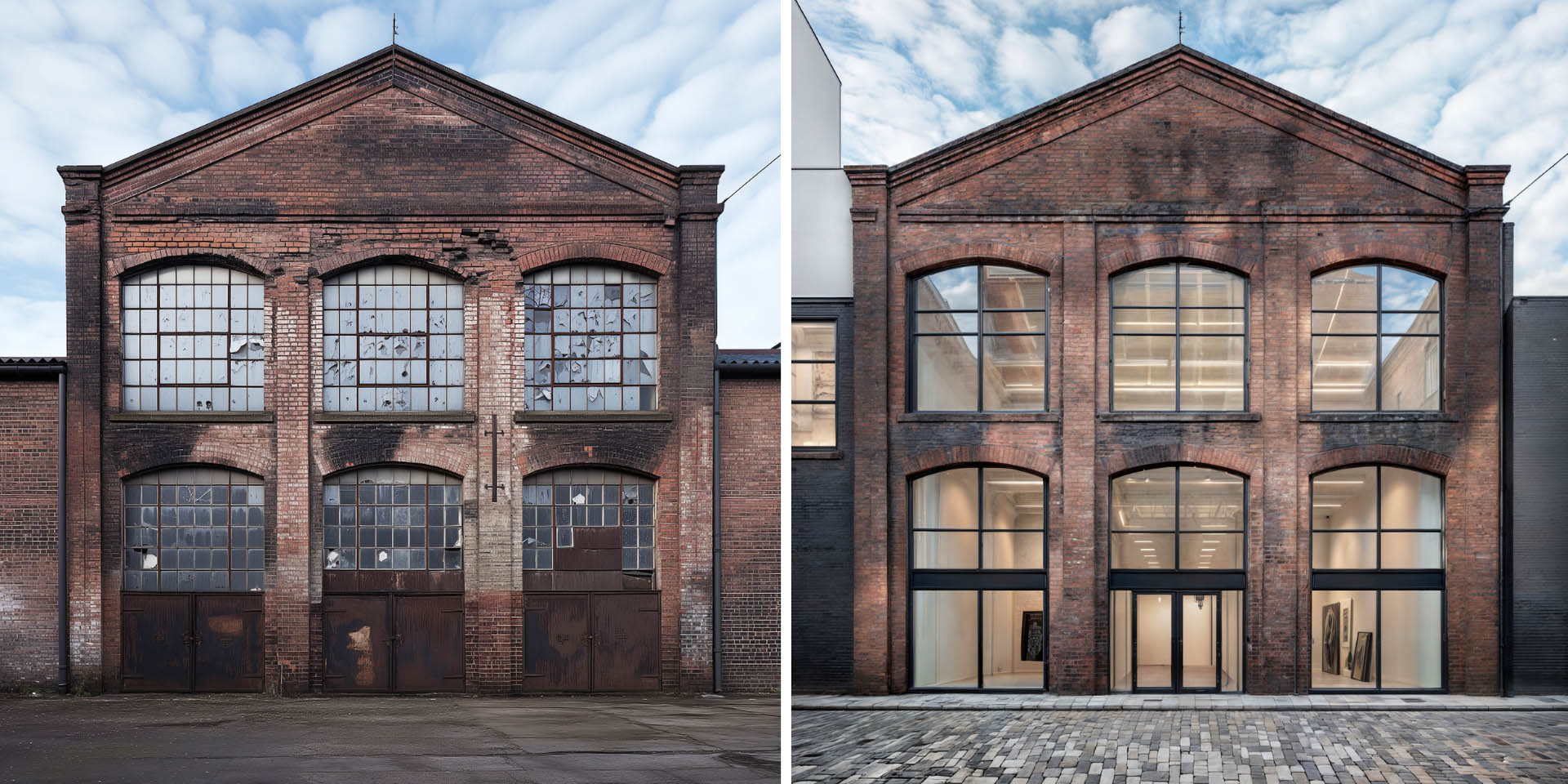
AI feels different
The introduction of GenAI to these very common practical design tasks is more akin to plugging in a synthesiser or effects-pedal into a musical instrument. In general, I prefer the “instrument” rather than “tool” analogy, which just conjures feelings of crude hammers and nails. By contrast, this instrument is nuanced, unpredictable and can make its own decisions.
My initial expectation about various AI image tools is that they would prove to be a like-for-like replacement for traditional digital rendering methods.
The reality is quite different; this is an entirely new angle with which to approach the same challenge and requires a fundamental shift in the way you think about things.
Indeed, this has been a source of debate between myself and Ismail Sileit, who says about Image Diffusion:
“While traditional rendering techniques are about faithfully replicating reality through precise algorithms, GenAI allows us to engage in a live dialogue with possibility. It’s not so much about rendering accuracy —it’s more about cultivating a relationship with the unpredictable, the emergent, and the profoundly novel.” Ismail Sileit, architect at Fosters + Partners and creator of form-finder.com (in a personal capacity)
What Ismail is getting at here is that yes — there are certainly time savings, but we shouldn’t overstate these. The main change he perceives is in the speed and breadth of the creative feedback loop itself, the new experimental avenues that we’re able to explore, and importantly the enhanced enjoyment of the whole process.
Images: what’s hard?
Despite what the “AI is easy” influencers tell you, it’s also not a simple process if you want to achieve results you can use on real projects – to exercise real control and achieve usable results in practice you have to learn quite complex interfaces and become familiar with a new lexicon of technical terms like; “denoising”, “latent space”, “seeds”, “checkpoints” and “LoRAs”, to name a few.
Getting the best out of image models also requires a strong dose of curiosity, patience, and a willingness to keep persisting in the face of abject ugliness at times.
When we’re working on this kind of thing our overall hit rate is probably less than 5%. For a recent feasibility study presentation using some very basic internal 3D renders to start things off, we produced 6no total new images across 3 design studies – but when I look back at the results, I can see it took us about 211 separate tests to get there.
While the study produced these images very rapidly, we had to put up with a great deal of mediocre and downright appalling outputs to find something that captured what we were looking for.
A 3-5% conversion doesn’t sound like a great hit rate, and also feels inherently wasteful. When you’re generating images, your GPU will be getting ready to take off and use a lot of electrical energy and this is an area I’m looking into in more detail currently to better understand the actual energy impact of using GenAI at any kind of scale. Any practice looking to adopt GenAI will probably also need to establish a means of measuring their energy use intensity and as model size increases in the coming years, this will probably become an ever greater area of difficulty.
The most effective AI strategies in professional practice that I’ve seen aren’t mandated from on high, but rather emerge organically from teams who are given the freedom to experiment and trust to exercise their individual judgement about what to do with the results
Text work in practice
AI image work really lights up the right side of my brain, but the AI technology that feels most important to me and that I utilise more than any other – by far – is Large Language Models (LLMs).
My use of text models is growing over time and I’m now at around 10-20 tasks a day for very varied and high-utility responses.
Early on I set GPT as my homepage and I tried to use it for as much as I could and even with this proactive attitude to adjust my working methods, I still spent months forgetting that it was there to help for all kinds of things during the day.
This behavioural change phenomenon may actually be the greatest hurdle in the short term to any kind of meaningful change because workflow muscle memory is strong and the cost of a failed effort during daily working life is high.
The table below shows just a few examples of the type of things we can now attempt with a fair expectation of success. With the latest release of models and the next wave just around the corner, the use-cases will be only growing in the coming months and years.
“Next-generation AI models are effectively embargoed until after the US election on 5th November, but expect to see significant gains in reasoning ability and intelligence when they arrive, with each of the main providers currently training / testing models more than an order of magnitude larger than the current largest & most-intelligent models.” Stephen Hunter, Buckminster AI
Text work. What’s hard?
There is no shortage of people expounding the virtues of LLMs, but let’s be honest about the difficulties.
“Multi needle reasoning” problems. These happen when you ask the model to retrieve a number of separate facts at the same time and then to apply further layers of reasoning and logic on top. When you request too many needles and too many processes, you may get a few okay results, but it’s possible to ask too much of the model at once and this can result in disappointing or incomplete results. There are ways around this that involve breaking down the task into smaller steps and just giving the model what it needs at each step, hence why you need to learn good prompt craft.
Accuracy. You still have to always spot check the results and validity of your workings. I call it spot check, because with the latest models if it’s set off on the right track it will usually get things right. Hallucinations remain a challenge, albeit the rate of occurrence has been dramatically reduced since I first started using them nearly two years ago, such that errors are increasingly hard to identify. This is why spot-checks must remain part of your core approach for now.
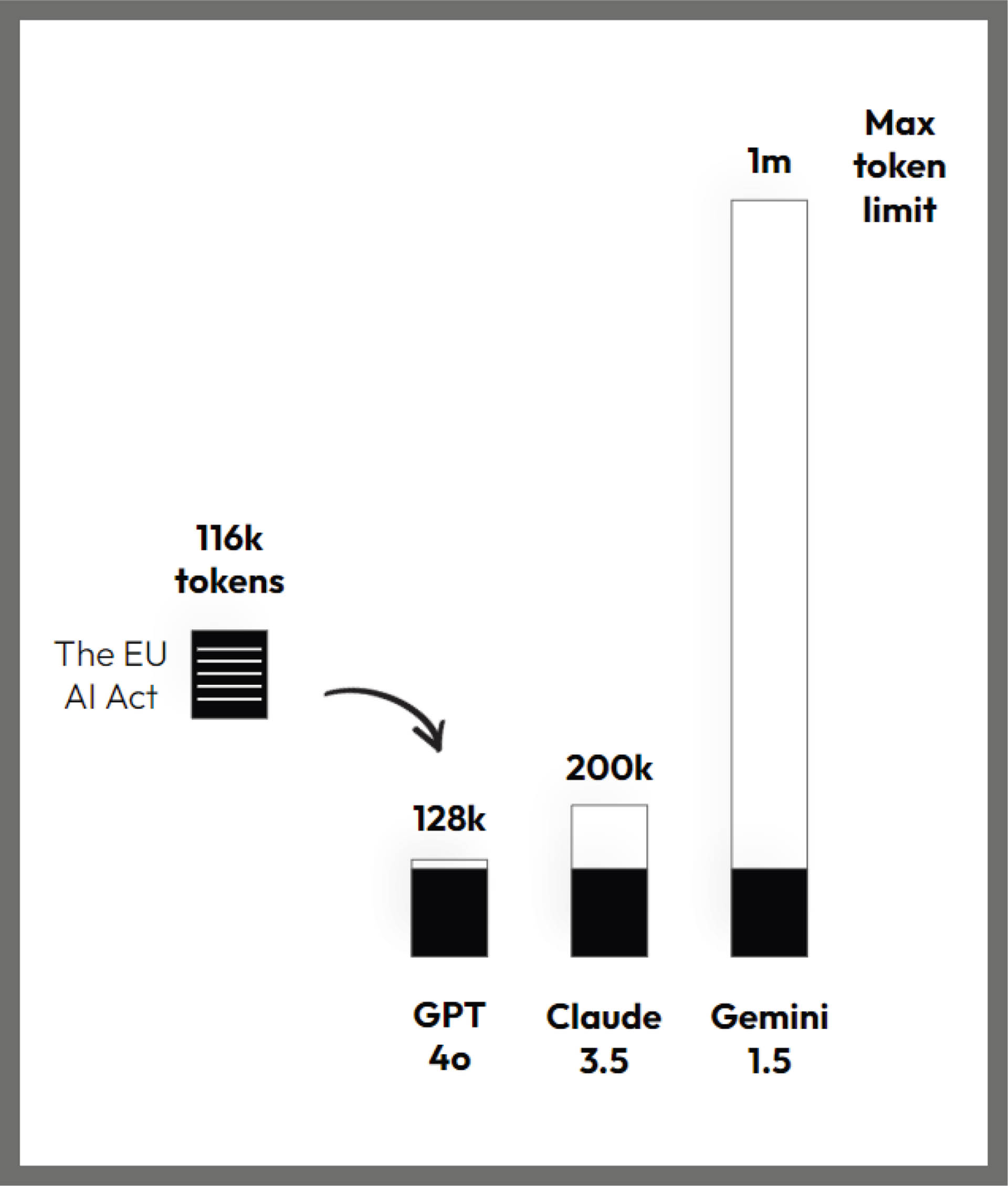
Long prompting is now better than using Custom GPTs for most tasks. Now that we have large context-window models available we can work with hundreds of thousands of words for input. For most tasks, pasting your prompts in long-form into a model is now a more robust approach than Custom GPTs if you need very reliable performance.
This is because Custom GPTs typically run on a smaller context window (32k) and also involve RAG, a process that cuts your data up into small chunks that are later retrieved, without needing to store them in full within the knowledge base. If the wrong chunk is retrieved, you will get a sub-par answer. Many practices have found use for Custom GPTs, but it’s now better to move towards a long-prompting method and make full use of these amazingly spacious context windows.
Data preparation. Most businesses are excited about the potential of AI, but they aren’t excited about preparing all their reusable project and operational data such that they can use it again and again to produce first draft written reports of many kinds. There is a surprising amount of written work in practice that could be massively aided by GenAI if this data were ready. We really need to start thinking about building asset libraries that are ready for LLMs to process and link together in new ways to really feel the benefits.
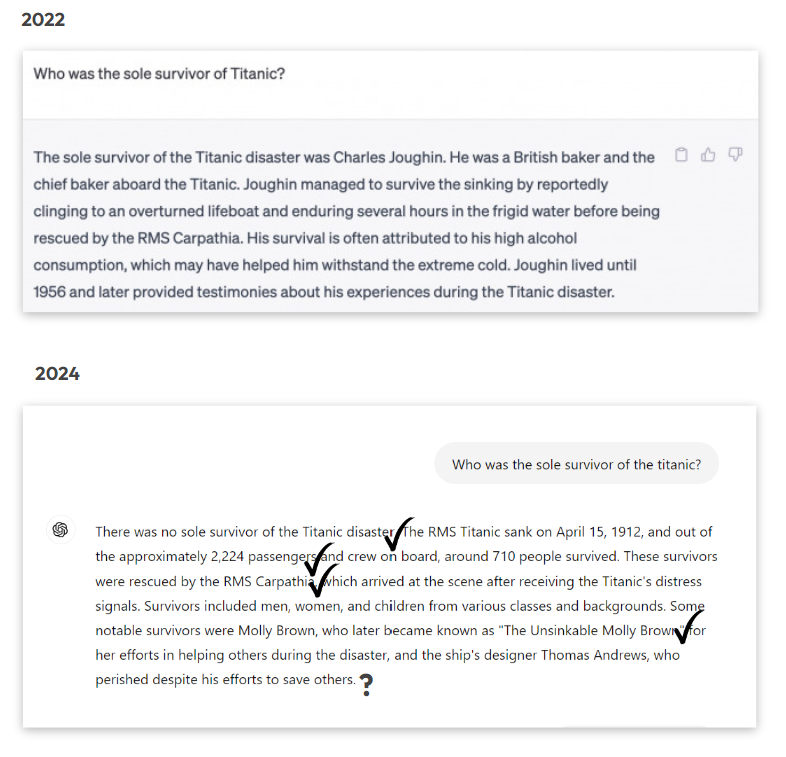
Conclusion – team-led AI
The most effective AI strategies in professional practice that I’ve seen aren’t mandated from on high, but rather emerge organically from teams who are given the freedom to experiment and trust to exercise their individual judgement about what to do with the results.
This team-led approach to AI adoption, where workers take responsibility for how they wish to use it, allows for a more nuanced integration that respects existing workflows while uncovering new efficiencies and enthusiasm from within the team.
Consider building a “heat map” of opportunities within your organisation. Where are the places that these ideas really fit without trying too hard? Once you’ve identified these hotspots, tackle them one at a time. Small, incremental changes often lead to the most sustainable transformations.
AI is neither a panacea nor a parlour trick – it’s a curious instrument that, when wielded with skill and discernment, can help us work smarter, faster, and perhaps even with greater enjoyment.
We are still so early. So, I urge you to withhold judgement; be experimental and be curious.
About the author
Keir is an architect operating with one foot in architectural practice and one in the development of Generative Design and AI tools and workflows. He founded the creative consultancy, Arka Works in 2023 following a directorship at AJ100 practice Morris+Company, with a mission to prepare the profession for AI-driven change. He does this by helping architects, clients and startups to effectively apply the latest Generative Design and AI tools to the work they already do in practice, so that they can adapt to a rapidly changing professional landscape.