Greg Corke caught up with Keith Bentley, CTO of Bentley Systems, to find out why he thinks machine learning is so important to digital twins and why we’ve only just scratched the surface
In 2018 Bentley Systems acquired machine learning and IoT development company AIworx. At the time, CTO Keith Bentley described it asan ‘acqui-hire’, ‘just for the talent’. After all, the Canadian firm didn’t actually have any products.
Two years later and it’s starting to become clear exactly why Bentley brought in that talent. The team has been playing a key role in developing new technologies for Bentley’s Digital Twin platform, as the company looks to establish itself as the leading force in this emerging sector.
According to Keith Bentley, the AIworx team has been split into two groups: one is looking at ways to make smarter, better quality input to the digital twin, while the other is trying to analyse the dynamics within the digital twin.
Feeding the twin
If all digital twin projects were created for brand new assets, then the development of a digital twin would be much simpler. The reality is, most digital twins are likely to be built for existing buildings or infrastructure, for which the quality and format of data can be extremely varied.
With this in mind, the AIworx team has been working out how to ‘up-level’ the content that goes into a digital twin, as Keith Bentley explains, “So we have paper, we have pictures, we have CAD files, and sometimes BIM models, and when you combine all that together, you find that there’s a lot of inconsistency.”
Keith Bentley highlights one AIworx project that automatically recognises all the tags on a scanned Piping and Instrumentation Diagram (P&ID) drawing, then relates that into the 3D model. Another example is the automatic object classification of reality capture meshes through analysing the input of photographs.
Smart thinking
Digital twins are fundamentally about big data: collecting old, new, live, and future data, geo-referencing it, finding out what has changed, displaying it, and doing something meaningful with the data. It’s in this last part that machine learning can be used to great effect, studying patterns in the data, to help AEC teams and infrastructure owners assess the impact of past decisions to help make more informed design, business and operational decisions moving forward.
Keith Bentley shared some specific examples of what the team are exploring, including studying what makes a good design or what makes something fail frequently by analysing maintenance records.
“Recognising patterns and being able to analyse the contents of a digital domain or the digital twin ecosystem, combining the design, the reality data, the IoT data, and trying to put that all together to give better informed kind of business input. You know, should your needle look red, if you’re headed for disaster or something like that. And there’s no end of that…”
Knowledge sharing
Of course, the more data that’s collected, the better the insight. A few years ago, Bentley floated the idea of engineering firms sharing analysis data from their projects so they could be compared against others. Could machine learning be used in this way for the greater good?
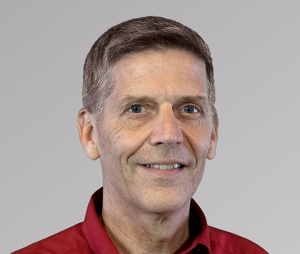
“When you get to things like trying to recognise P&ID documents, there’s a lot of patterns that are the same across everybody’s P&ID,” explains Keith Bentley. “So, if you could study the Universe of all P&IDs and have that go into the model, the results would be way better.
“Asking people ‘hey, would you be willing to donate the analysis of your data into the machine learning model that will then give back to you?’ that hasn’t happened yet, but I still hold out hope that people will be motivated by that, if we could show them value at the other end.
“But I have to confess so far, it’s just we do it on each user’s data in isolation.”
The future
It’s still very early days for the use of machine learning in infrastructure digital twins, especially when it comes to recognising patterns. There have been several examples of the technology used for predictive maintenance, but in reality, we’ve only just scratched the surface.
Keith Bentley explains that one of the reasons it hasn’t happened yet is that the data just doesn’t exist or isn’t available. But this will change over time.
“Ten years from now, all the demos will be about how smart your digital twin is – smart, meaning your machine learning can recognise patterns in your data that you didn’t know were there,” he says.
“Digital Twins will be the input system to the machine learning system that I think can revolutionise the concept of designing, operating, building, and maintaining large scale assets in the decades to come.”
If you enjoyed this article, subscribe to our email newsletter or print / PDF magazine for FREE